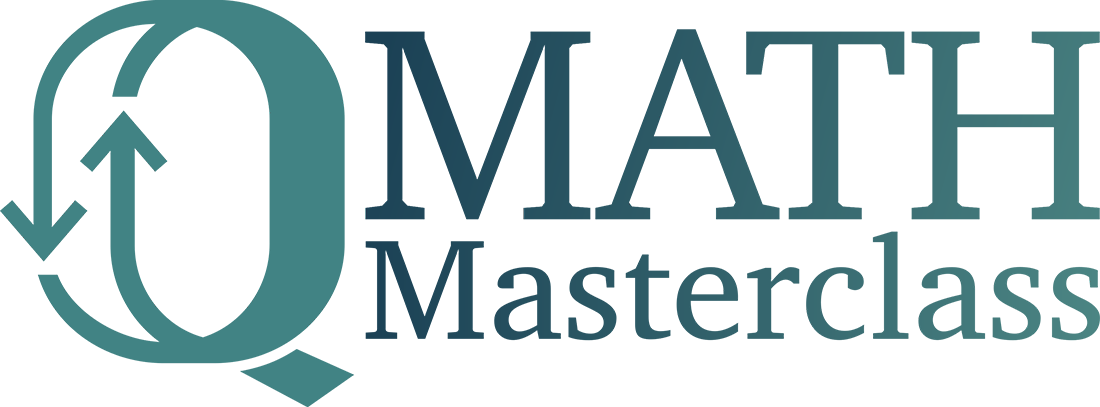
Quantum Learning Theory
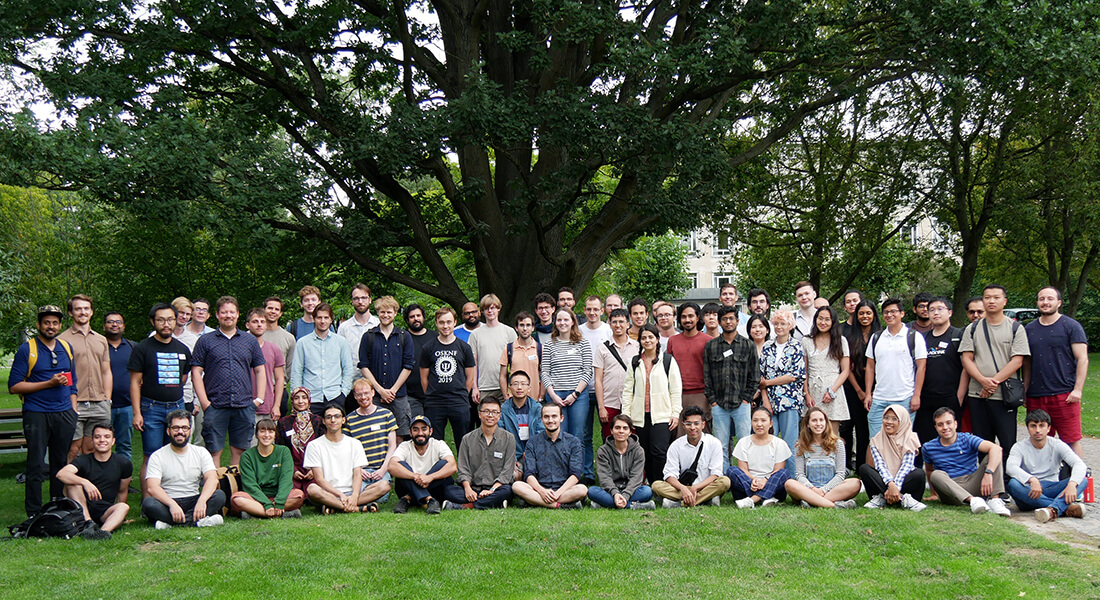
August 21-25, 2023
We are entering the exciting era where quantum computers are no longer a theoretical construction but a physical reality. However, now and in the near future, the available quantum computing hardware is noisy. Therefore, it is crucial to use quantum resources efficiently: the difference between noise and signal may lie in just a slightly smarter use of the number of available qubits or circuit depth. A convenient theoretical perspective is provided by quantum learning theory, which poses the following question: given access to a quantum state, how can we learn properties of the state as efficiently as possible?
Apart from using near-term noisy devices for useful applications, quantum learning theory is also fundamental to understand the power of quantum computation for future fault-tolerant devices. How does quantum learning compare to classical learning theory? Can we find speed-ups over classical algorithms when trying to learn either classical or quantum systems?
Target audience
This master class will be of interest for all students and early career researchers interested in properties, applications and future research directions involving quantum learning theory.
Location
The master class will take place at the University of Copenhagen, Denmark. Copenhagen is a beautiful harbor city, famous for being among the safest and most enjoyable places to live in the world.
Participants of the master class will have the opportunity to explore the city from the water during an excursion. August is the second warmest month of the year in Copenhagen with about 14 hours of daylight.
We are happy to have the following leading experts in quantum learning theory to give lectures in the school:
- Jonas Helsen (CWI & QuSoft)
- Hsin-Yuan (Robert) Huang (Caltech)
- Yihui Quek (Harvard)
- Ingo Roth (TII)
- Ronald de Wolf (CWI, University of Amsterdam & QuSoft)
These will be supplemented by the speakers:
Schedule QMATH masterclass 2023 | |||||
Mo | Tue | Wed | Thu | Fri | |
9:00 – 10:40 | Registration + coffee | Ronald de Wolf | Jonas Helsen | Ingo Roth | Robert Huang |
10:40 – 11:00 | Welcome | Coffee break | |||
11:00 – 12:40 | Ronald de Wolf | Ingo Roth | Yihui Quek | Jonas Helsen | Yihui Quek |
12:40 – 14:00 | Lunch | Open problem lunch | |||
14:00 – 15:40 | Laura Mancinska | Free/canoe excursion | Ronald de Wolf | Robert Huang | |
15:40 – 16:00 | Coffee break | Coffee break | |||
16:00 – 17:00 | Ingo Roth | Tim Möbus | 16:30 Poster and pizza | ||
17:15 Welcome reception | 19:00 Social dinner |
Ronald de Wolf: Quantum learning theory: samples, time, queries
Quantum learning theory studies the theoretical aspects of how quantum computing can change and improve machine learning. I will introduce several mathematical models of learning, which can be instantiated to classical or quantum data: the Probably Approximately Correct (PAC) model and exact learning from membership queries. We will see to what extent quantum computers can improve the sample complexity and/or the time complexity of learning in these models. My three lecture blocks will cover:
1. The PAC model of learning theory. Learning from quantum examples. Positive results using Fourier sampling.
2. Sample complexity of quantum PAC learning. Learning from quantum membership queries.
3. Time complexity of quantum PAC learning.
Reading material:
I will mostly follow my survey paper with Srinivasan Arunachalam: https://arxiv.org/abs/1701.06806
For general background on quantum computing (especially algorithms and complexity), you could have a look at: https://arxiv.org/abs/1907.09415
Yihui Quek: information-theoretic lower bounds for learning
Science is often seen as a pursuit to expand the boundaries of what can be done: to develop new algorithms and insights; to learn faster and better. We know far less about what we can’t do. And yet, knowing the boundaries of what is possible would save us from wasting effort to further optimize our solutions beyond that point; more importantly, proving a lower bound often yields deeper insights into the nature of measurements and quantum computation itself.
After a brief tour through common techniques to design sample efficient learning algorithms (also known as upper bounds), I will delve into information-theoretic lower bounds for learning and statistical inference, including the ONE cool reduction that instantly makes a learning lower bound into an information-theoretic exercise! If time permits, we can also take a foray into computational lower bounds.
Robert Huang: quantum advantages in learning from experiments
Quantum technology has the potential to revolutionize how we acquire and process experimental data to learn about the physical world. A quantum learning agent that transduces data from a physical system to a stable quantum memory and processes that data using quantum computation could have significant advantages over classical learning agents, which measure the physical system to obtain classical data and process the data using classical computation.
This lecture presents a mathematical framework for proving that quantum agents can learn from exponentially fewer experiments than classical agents. We will look at multiple tasks with an exponential quantum advantage, such as predicting many incompatible properties in physical systems, performing quantum principal component analysis, learning approximate models of physical dynamics, and distinguishing different kinds of evolutions. For each task, we will design an efficient algorithm for the quantum agent and prove that all algorithms the classical agent could run are exponentially inefficient.
References:
A general overview and introduction to proving quantum advantages in learning from experiments: Huang, Hsin-Yuan, et al. "Quantum advantage in learning from experiments." Science 376.6598 (2022): 1182-1186. arXiv link: https://arxiv.org/abs/2112.00778
More advanced mathematical/conceptual techniques for proving quantum advantages in learning: Chen, Sitan, et al. "Exponential separations between learning with and without quantum memory." 2021 IEEE 62nd Annual Symposium on Foundations of Computer Science (FOCS). IEEE, 2022. arXiv link: https://arxiv.org/abs/2111.05881
The quantum learning algorithm for predicting all 4^n Pauli observables from only O(n) samples of the unknown state \rho is given in this work: Huang, Hsin-Yuan, Richard Kueng, and John Preskill. "Information-theoretic bounds on quantum advantage in machine learning." Physical Review Letters 126.19 (2021): 190505. arXiv link: https://arxiv.org/abs/2101.02464
Ingo Roth: Quantum device characterization and tomography
Quantum device characterization is the central application of quantum learning theory in today's experiments. The precise control of complex quantum systems promises numerous technological applications including analogue simulation and digital quantum computing. The complexity of such devices renders the characterization and certification of their correct functioning a challenge. To address this challenge, numerous methods were developed in the last decade.
In this masterclass, we provide an introduction to the field of quantum device characterization and its mathematical foundations, focusing on one of the still most frequently used techniques in practice: quantum state tomography.
Laura Mancinska: Certification of large quantum systems.
In this talk I will introduce the concept of self-testing which aims to answer the fundamental question of how do we certify proper functioning of black-box quantum devices. Self-testing is the strongest form of certification and it allows a classical user to deduce the quantum state and measurements used to produce certain measurement statistics. The main goal of this area is to understand which states and measurements can be certified and how efficiently.
We will see that surprisingly it can be possible to certify arbitrarily large quantum states and measurements with just four binary-outcome measurements. I will also present a general method for certification of quantum measurements. We will see a scheme that allows us to self-test an arbitrary real projective measurement.
Tim Möbus: Dissipation-enabled bosonic Hamiltonian learning via new information-propagation bounds
Reliable quantum technology requires knowledge of the dynamics governing the underlying system. This problem of characterizing and benchmarking quantum devices or experiments in continuous time is referred to as the Hamiltonian learning problem. Recently, bosonic systems have gained significant attention in continuous quantum error correction in forms of the cat code implemented by superconducting circuits. In contrast to multi-qubit systems, learning guarantees for the dynamics of bosonic systems have hitherto remained mostly unexplored.
In this talk, I will explain our learning algorithm for multi-mode Hamiltonians given as polynomials in annihilation and creation operators with modes arranged on a lattice (see arXiv: 2307.15026). To tackle the intrinsic unboundedness of the annihilation and creation operator, we establish a simple moment criterion in terms of the particle number operator which ensures that learning strategies from the finite-dimensional setting extend to the bosonic setting, requiring only coherent states and heterodyne detection on the experimental side.
The field of quantum learning has seen explosive growth in recent years. Three developments that will be extensively covered in this masterclass are:
- Proofs of separations where efficient classical learning is impossible, while quantum learning can be efficient. There are examples where the learning problem is quantum mechanical, and the goal is for instance to learn in which phase a quantum state of matter is. On the other hand, there are also results showing that in certain situations there is no strong separation between quantum and classical learning.
- The method of shadow tomography, where certain properties of quantum states and processes can be estimated from a surprisingly small number of copies. The idea is that one can perform certain random measurements, and extract expectation values of observables from these measurements. These results appear to be very promising to significantly reduce resources for quantum computation.
- The use of advanced methods, such as randomized benchmarking, to analyze noise in quantum devices. This is an important tool in improving quantum computing hardware: as the quantum computer increases in size, it becomes harder to fully characterize the noise processes that affect it, and naïve tomography would be too inefficient.
A detailed description of the program, with abstracts for the lectures of each of the speakers will appear later.
We kindly ask the participants to arrange their own accommodation.
A good option can be Hotel 9 Små Hjem, which is pleasant and inexpensive and offers rooms with a kitchen. Other alternatives are Steelhouse Copenhagen and CabInn, which has several locations in Copenhagen: the Hotel City (close to Tivoli), Hotel Scandivania (Frederiksberg, close to the lakes), and Hotel Express (Frederiksberg) are the most convenient locations; the latter two are 2.5-3 km from the math department. Somewhat more expensive – and still recommended – options are Hotel Nora and Ibsen's Hotel.
An additional option is to combine a stay at the CabInn Metro Hotel with a pass for Copenhagen public transportation (efficient and reliable). See information about tickets & prices.
The deadline for funding applications was June 30th. You will not receive a confirmation of acceptance before the application deadline.
If you are not applying for funding, please register by July 31st at the latest. Registration is now closed.
There is no registration fee. We do not cover travel funding.
The master class is organized by the Villum Centre for the Mathematics of Quantum Theory (QMATH) at the Department of Mathematical Sciences. The local organizers are:
Freek Witteveen
Any questions about the master class can be directed to Suzanne Andersen at suzanne@math.ku.dk
Deadlines
The deadline for funding applications is June 1st.
If you are not applying for funding, please register by June 15st at the latest.